A new world is opening to us now. This new paradigm creates exitment and fear at the same time. We all want to be part of this new technological revolution. We are focusing on how to create value in the Supply Chain Digitalization.
- Which elements of the Supply Chain can be improved?
- Which tasks can be processed by AI applications?
- What are the risks of NLP Natural Langage Processing?
Leveraging artificial intelligence (AI) capabilities in the Supply Chain can significantly enhance efficiency, visibility, and decision-making in the aerospace industry. Here are several ways AI can be applied to support the digital transformation of the supply chain
- Demand Forecasting:
- Use AI algorithms to analyze historical data, market trends, and external factors to improve demand forecasting accuracy. This helps optimize inventory levels and reduces the risk of stockouts or overstock situations.
- Predictive Maintenance:
- Implement AI-powered predictive maintenance models to forecast when aerospace components or equipment are likely to fail. This minimizes downtime, extends the lifespan of assets, and improves overall maintenance planning.
- Supply Chain Visibility:
- AI can enhance real-time visibility across the supply chain by analyzing data from various sources. This includes monitoring the status of shipments, predicting potential disruptions, and providing proactive alerts.
- Intelligent Sourcing:
- Apply AI to evaluate suppliers based on historical performance, quality, and other relevant factors. This helps optimize supplier selection and negotiation processes.
- Autonomous Vehicles and Drones:
- Explore the use of autonomous vehicles and drones for last-mile delivery, inventory management, and warehouse operations. AI enables these devices to navigate and make decisions in complex environments.
- Robotics and Automation:
- Integrate AI-driven robotics into manufacturing and warehouse processes. This includes automating repetitive tasks, such as picking and packing, to improve efficiency and reduce operational costs.
- Natural Language Processing (NLP):
- Implement NLP capabilities to analyze unstructured data, such as emails, documents, and customer feedback. This helps in gaining insights into market trends, supplier relationships, and customer demands.
- Blockchain for Transparency:
- Utilize blockchain technology with AI to enhance transparency and traceability in the supply chain. This is particularly crucial in aerospace, where regulatory compliance and the authenticity of parts are critical.
- Supplier Risk Management:
- Develop AI models to assess and mitigate supplier risks by monitoring geopolitical events, economic indicators, and other factors that may impact the supply chain.
- Dynamic Pricing Optimization:
- Use AI algorithms to dynamically adjust pricing based on market demand, competitor pricing, and other variables. This ensures competitive pricing strategies while maximizing profitability.
- Customs and Trade Compliance:
- Employ AI to navigate complex customs and trade compliance regulations. This reduces the risk of delays and penalties associated with non-compliance.
- Collaborative Robots (Cobots):
- Implement cobots that work collaboratively with human workers in manufacturing and assembly processes. AI helps these robots adapt to dynamic environments and perform tasks that require flexibility and precision.
- Energy Efficiency:
- Apply AI algorithms to optimize energy consumption in manufacturing and transportation processes, contributing to sustainability goals.
- Continuous Improvement:
- Implement AI-driven analytics to continuously analyze and optimize supply chain performance. This includes identifying areas for improvement, reducing inefficiencies, and enhancing overall productivity.
Implementing these AI applications requires a strategic approach, collaboration with technology partners, and a commitment to ongoing innovation. Integrating AI into the aerospace supply chain contributes to a more agile, responsive, and resilient ecosystem.
NLP can process the following tasks
Natural Language Processing (NLP) encompasses a variety of techniques and methods aimed at enabling computers to understand, interpret, and generate human language. The field of NLP is diverse, and different tasks within NLP can be categorized into several types. Here are some common types of Natural Language Processing tasks:
- Text Classification:
Sentiment Analysis: Determining the sentiment or emotional tone expressed in a piece of text (e.g., positive, negative, neutral).
Topic Classification: Categorizing text into predefined topics or categories.
- Named Entity Recognition (NER):
Identifying and classifying entities (e.g., names of people, organizations, locations) mentioned in a text.
- Machine Translation:
Translating text from one language to another automatically.
- Speech Recognition:
Converting spoken language into written text.
- Information Retrieval:
Retrieving relevant information from a large dataset based on user queries or search terms.
- Question Answering:
Developing systems that can understand and answer questions posed in natural language.
- Text Summarization:
Generating concise and coherent summaries of longer texts.
- Text Generation:
Creating human-like text based on given prompts or contexts.
- Coreference Resolution:
Resolving references to entities in a text and determining which words or phrases refer to the same entity.
- Word Embeddings:
Representing words as vectors in a continuous vector space, capturing semantic relationships between words.
- Syntax and Parsing:
Analyzing the grammatical structure of sentences, including part-of-speech tagging and parsing.
- Semantic Role Labeling (SRL):
Identifying the roles of different words or phrases in a sentence, such as the subject, object, or predicate.
- Text Clustering:
Grouping similar documents or sentences into clusters based on their content.
- Dialogue Systems:
Building systems that can engage in natural language conversations with users.
- Information Extraction:
Extracting structured information from unstructured text, such as relationships between entities.
- Paraphrase Detection:
Identifying whether two pieces of text convey the same meaning, even if the wording is different.
These tasks often involve the use of machine learning techniques, including supervised and unsupervised learning, as well as deep learning approaches like recurrent neural networks (RNNs) and transformer models. Advances in NLP are frequently driven by the development and refinement of neural network architectures and the availability of large, annotated datasets.
What are the risks of NLP?
- Ethical and Bias Concerns:
NLP models can inherit biases present in training data, leading to biased or unfair outcomes and potentially perpetuating existing societal biases.
- Lack of Explainability:
Many NLP models, especially complex ones like deep neural networks, can be challenging to interpret, making it difficult to explain how decisions are reached.
- Privacy Issues:
NLP systems may inadvertently reveal sensitive information, posing privacy concerns, especially when processing personal or confidential data.
- Security and Adversarial Attacks:
NLP models are susceptible to adversarial attacks where malicious actors intentionally manipulate input data to deceive the model and produce incorrect results.
- Misinterpretation of Context:
NLP models may struggle to understand nuanced context, leading to misinterpretations, particularly in cases where cultural or contextual nuances play a significant role.
- Generalization Challenges:
NLP models may have difficulty generalizing to new or uncommon language patterns or expressions not present in the training data.
- Dependency on Training Data Quality:
The performance of NLP models heavily relies on the quality and representativeness of the training data. Biases or inaccuracies in the data can impact the model’s effectiveness.
- Limited Understanding of Sarcasm and Humor:
NLP models may struggle to grasp the nuances of sarcasm, humor, or other forms of figurative language, leading to misinterpretations.
- Cultural and Linguistic Biases:
Biases present in training data may reflect cultural or linguistic biases, causing the model to favor certain demographics or linguistic groups.
- Regulatory Compliance and Legal Risks:
The use of NLP in certain applications may raise legal and regulatory challenges, particularly regarding data protection, privacy, and compliance with industry-specific regulations.
- Limited Context Understanding:
Some NLP models may lack the ability to understand broader context, resulting in responses that are contextually inappropriate or incorrect.
- Inadequate Handling of Ambiguity:
NLP systems may struggle to handle ambiguous language or situations, leading to uncertainty in their responses.
- Deployment Challenges:
Integrating NLP models into real-world applications may face challenges related to compatibility, scalability, and the need for continuous improvement.
- Social and Economic Impact:
The widespread use of NLP in certain applications may have social and economic consequences, such as job displacement and changes in communication dynamics.
Addressing these risks requires ongoing research, ethical considerations, transparency, and continuous monitoring and improvement of NLP models. Responsible development and deployment practices are essential to mitigate potential negative impacts and ensure the ethical use of NLP technologies.
Examples of AI generated images (Midjourney)

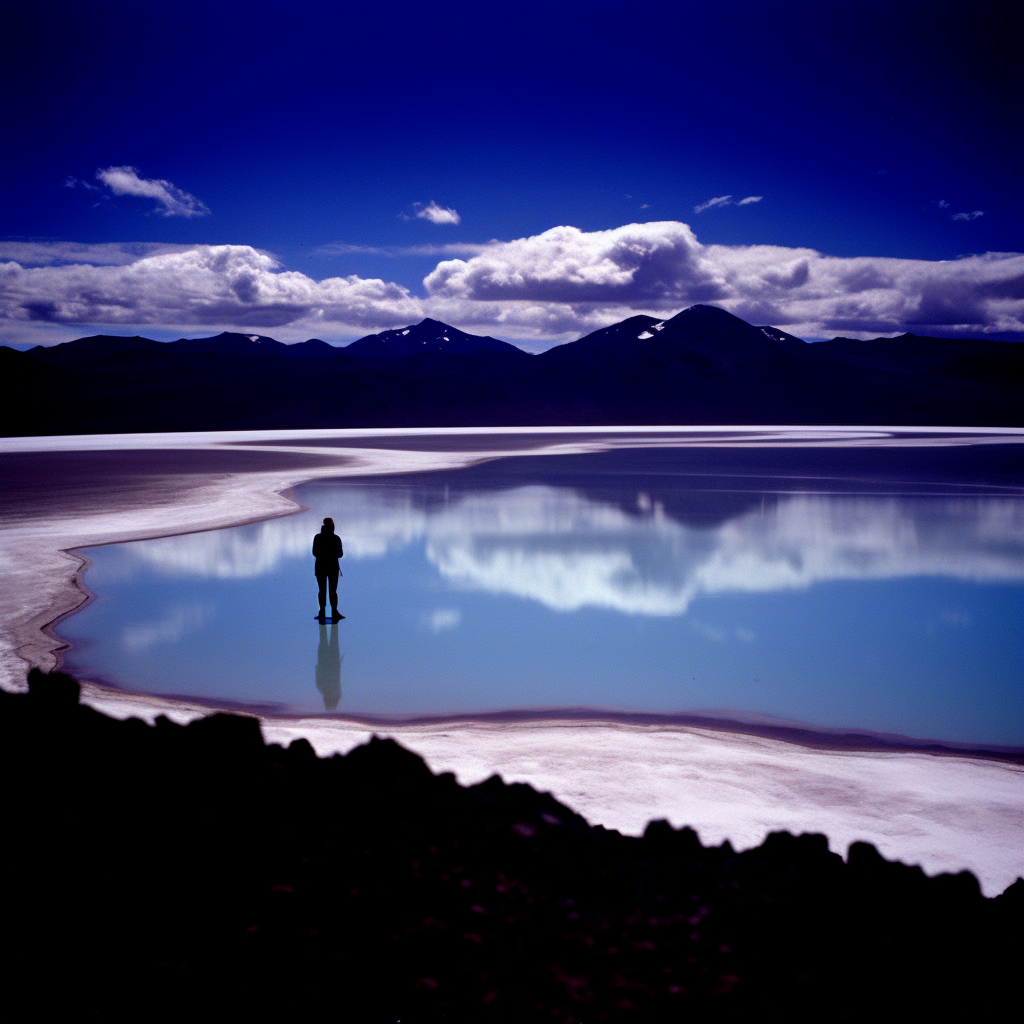
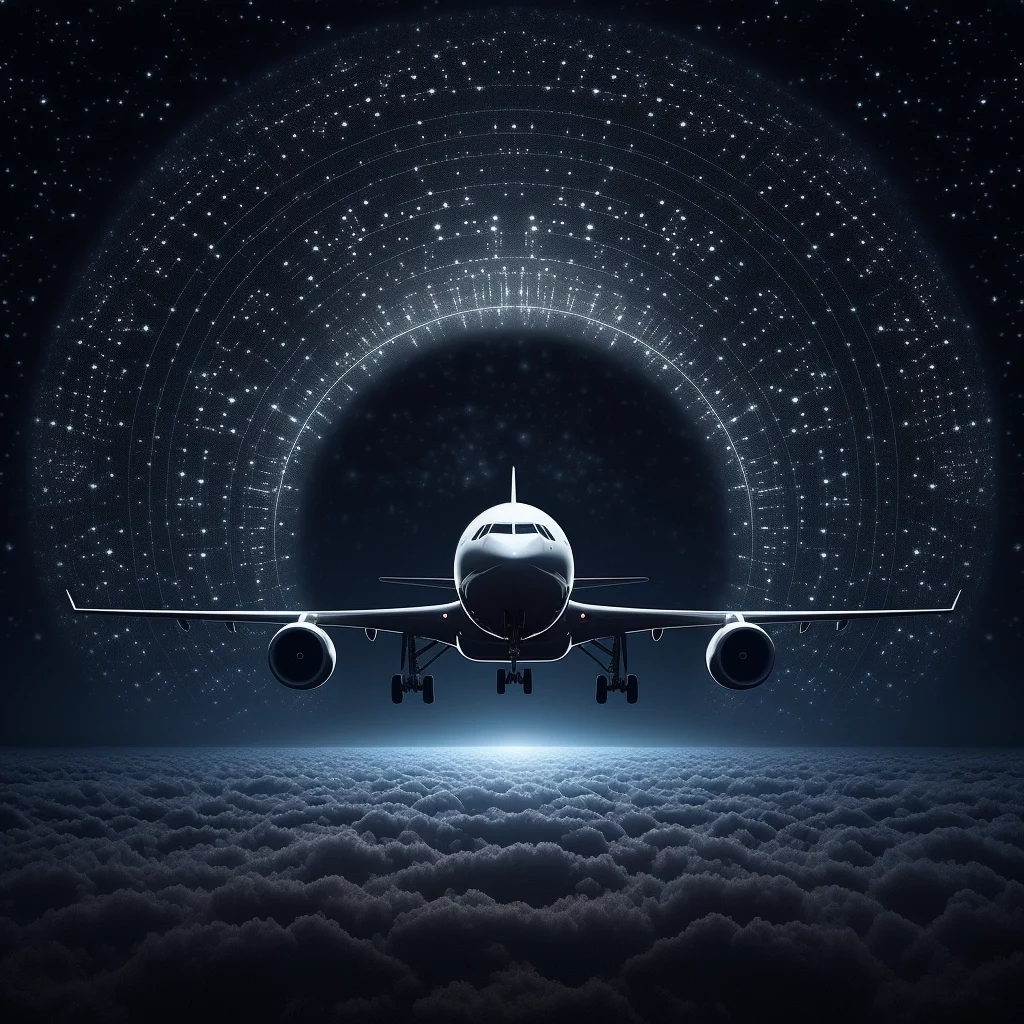